About me
I am a PhD student at Université de Montréal and MILA (Quebec AI Institute) under supervision of Aaron Courville.
I am currently working on generative models, with a particular focus on text-to-image systems. My research explores generation fidelity and diversity, aiming to develop responsible AI algorithms.
Keywords: Generative models, Multi-modality, Responsible AI, Fairness, Diversity, etc.
News
-
Recipient of Scholarship!
March 2025
I am grateful to Mila for supporting my research through the EDI in research scholarship.
-
Meetup at Neurips!
Dec. 2024
I will attend NeurIPS 2024 in Vancouver, presenting my work on bias analysis in unconditional image generative models!
-
Join Meta!
Sep. 2024
I joined FAIR, Meta, as a Visiting Researcher!
-
EMNLP 2023
Nov. 2023
One paper (Pre-trained Model Selection) is accepted to the Findings of EMNLP 2023!
-
ICLR 2023
Jan. 2023
One paper (Knowledge Update and Model Editing for LLMs) is accepted to ICLR 2023!
-
EMNLP 2022
Sep. 2022
Two papers (MoE Attention architecture, Prompt Learning and Meta Learning) are accepted to EMNLP 2022!
Contact
MILA (Quebec AI Institute),
6666 Rue Saint-Urbain,
Montréal, QC H2S 3H1, Canada
xiaofng [DOT] zhang [AT] gmail [DOT] com
Potential Chat
I'm happy to connect with YOU. If you want to chat with me, please look at my available time slots in the following Google Calendar. You could directly send me an Google Calendar invite via my gmail address stating about what topic you would like to talk.
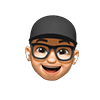